Today’s business leaders collect data at regular intervals from source systems like ERP applications to collect company information. When this data is migrated to a certain data warehouse, data quality is enhanced by organizing and enriching with data from other sources. Further, this data warehouse becomes the main source of information for reporting and analysis and can be used for dashboards.
Identify the need for a Data Warehouse
Many organizations fail in deploying a data lake due to lack of capability to identify a clear business case for it. Organizations that identify business challenges for their data and stay focused on finding the right solution, are more likely to remain well-positioned for success. We’ve listed below a set of key reasons why businesses might need a data warehouse service:
1. Standardize your data
Standardizing your data gathered from different sources improves operational accuracy and lessens the risk of possible errors. It becomes easier for business leaders to analyze data stored in a standard format making and gain actionable insights.
2. Enhance decision-making
A lot of businesses make decisions without proper analysis and having a clear picture of data, while well-established businesses develop data-driven plans and strategies. Data warehousing enhances the speed and operational efficiency of data access, enabling business leaders to gain a competitive edge.
3. Reduce costs
Data warehouses enable business users to evaluate the outcome of past initiatives based on historical data insights. They use strategic business ways to increase work efficiency, reduce costs and effort, generate revenue, thereby improving the bottom line.
Data Warehouse vs. Data Lake
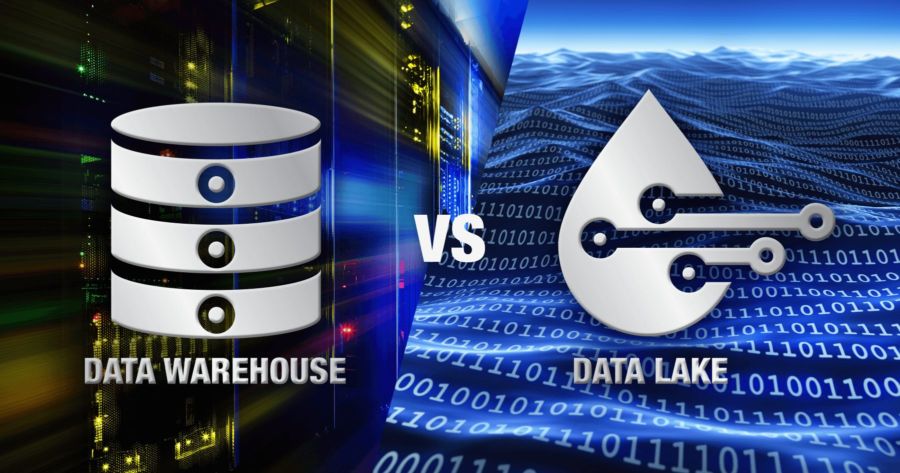
1. Data Warehouse
Data Warehouse is defined as collection and storage of data from multiple disparate data sources that includes organizations’ operational databases and external systems alike. A data warehouse generally stores structured data and supports the analytics needs of a business. It supports a fixed processing business strategy and is best-suited for certain use cases and complex requirements.
2. Data Lake
A data lake refers to collection of raw and unorganized data from disparate data sources. It supports exploratory analysis and various processing approaches like machine learning, heavy batch competition, data discovery, etc.
Data Warehouse | Data Lake | |
Objective of Data | The reason for storing data is pre-defined | The reason for storing data is undefined |
Structure of Data | Comprises of structured or processed data | Comprises of raw or unstructured data |
Users | Used by business users | Used by data scientists |
Accessibility | Extremely complex and making modifications can be expensive | Easily accessible and can be updated quickly |
Maturity | Strong maturity model | Emerging technology |
3. Agile Approach
It can take quite a while to build a modern data architecture, depending on how complex data is. During the data warehouse implementation, businesses usually cannot realize the value of investment resources. Over time, business requirements evolve and vary from the initial stage. Following an agile approach reduces the risk of failure by enabling the data warehouse to focus on business challenges and get more advanced as business requirements evolve.
An agile business model is an iterative process that involves development of modern data warehouses and business users throughout the process to provide continuous feedback. Agile data warehouse provide quick results compared to the traditional big bang approach.
4. Analyze and Understand Your Data
A data warehouse is a central repository to collect information from a wide range of sources. The data stored in a data warehouse should be clean, precise, and consistent to extract its maximum value. Thus, it is crucial to identify all the data sources and understand the features of all possible data sources.
Ideally, all the information is generated from an integrated, enterprise-wide data architecture. This approach reduces the time, effort, and costs associated with building & maintaining a data warehouse. Also, it enhances the quality of data in the data warehouse.
5. Analyze How Frequently You Need to Load Data
Batch processing is an efficient way for processing massive volumes of data when a number of transactions are collected over a span of time. Data is collected, analyzed, processed, and then the batch results are generated. It helps organizations cut operational expenses as it doesn’t require data entry personnel to support its functioning.
On the contrary, real-time data processing involves a continual input, process, and data output. While batch-processing is best-suited for most organizations, some organizations require real-time data processing for some use cases. With real-time data processing and analytics, organizations can take meaningful actions at the right time.
6. Define a Change Data Capture (CDC) Policy for Real-Time Data
The CDC policy enables organizations to capture any changes implemented in a database and ensure these changes are replicated in the data warehouse. The changes are tracked and stored in relational tables generally known as change tables. These change tables enable a historical data view changed over time.
CDC is a highly efficient mechanism to reduce the impact on source while loading new data into the data warehouse. This eliminates the need for bulk load updating and optimizes your data migrations.
7. Preference for ELT Tools instead of ETL
ELT and ETL are termed as two of the common practices for collecting data from multiple sources and storing in a data warehouse. Generally, data warehouses use either of the two common practices for data integration. Unlike ETL, the competitive advantage of ELT is the ease of storing unstructured data and flexibility. With ELT, users can store all types of information and help BI analysts save time while dealing with new information.
8. Select On-Premise or in the Cloud
A data warehouse integrates business data from cloud applications, serving as a centralized repository to support decision-making. Many organizations are preferring cloud-based alternatives to offer better governance and regulatory compliance. Cloud-enabled data warehouses offer on-demand scalability and ensure financial & operational efficiency with added capabilities such as identity & access management.
Find out the best practices of building a cloud-based modern data warehouse practice to produce real-time, reliable, and quality data for all your business units.
You may also read: 11 Online Marketing Strategies That Will Get You Results