In the fast-paced digital era, where online transactions have become the norm, there is an alarming rise in payment fraud incidents. The threat of fraud looms over individuals, businesses, and the entire economy, making implementing robust measures to protect against these malicious activities imperative. Thankfully, advancements in the technology of a fraud management system, particularly in machine learning, have paved the way for more effective credit card fraud detection and prevention.
In this article, we delve into the pivotal role that machine learning algorithms play in the ongoing battle against payment fraud. Machine learning, a branch of artificial intelligence, equips us with the ability to analyze vast volumes of transaction data, identifying hidden patterns and anomalies that might indicate fraudulent behavior.
It is a vigilant guardian, tirelessly sifting through data to safeguard our digital transactions.
Understanding Payment Fraud
To grasp the significance of machine learning in detecting and preventing payment fraud, it is crucial to develop a comprehensive understanding of the various forms that fraud can assume. Payment fraud encompasses a range of deceptive tactics fraudsters employ, targeting individuals and businesses. By familiarizing ourselves with these fraudulent schemes, we can better appreciate the importance of leveraging advanced technologies to combat them effectively.
One prevalent form of payment fraud is account takeover, where fraudsters gain unauthorized access to a user’s account, often through phishing or credential theft. Once inside, they exploit the account for personal gain, conducting unauthorized transactions or stealing sensitive information. Another notable form of payment fraud is card-not-present fraud, which occurs when unauthorized transactions are made without physically presenting the payment card. This fraud commonly transpires in e-commerce transactions, where fraudsters exploit stolen credit card information to make purchases.
Traditional approaches to fraud prevention often fall short in combating the evolving tactics employed by fraudsters. This is where machine learning assumes a pivotal role. By employing machine learning algorithms to analyze vast volumes of transaction data, we gain a significant advantage in identifying patterns, anomalies, and indicators of fraudulent behavior. Machine learning acts as a tireless sentinel, continuously monitoring transactions and detecting subtle signals that could signify fraudulent activities.
Machine Learning for Credit Card Fraud Detection
Machine learning algorithms form the backbone of an effective fraud management system, empowering us to combat payment fraud more accurately and efficiently. These algorithms can analyze vast volumes of transaction data, extracting valuable insights and patterns that human analysts may overlook. By harnessing the power of machine learning, we can perform proactive credit card fraud detection, bolstering the security of digital transactions.
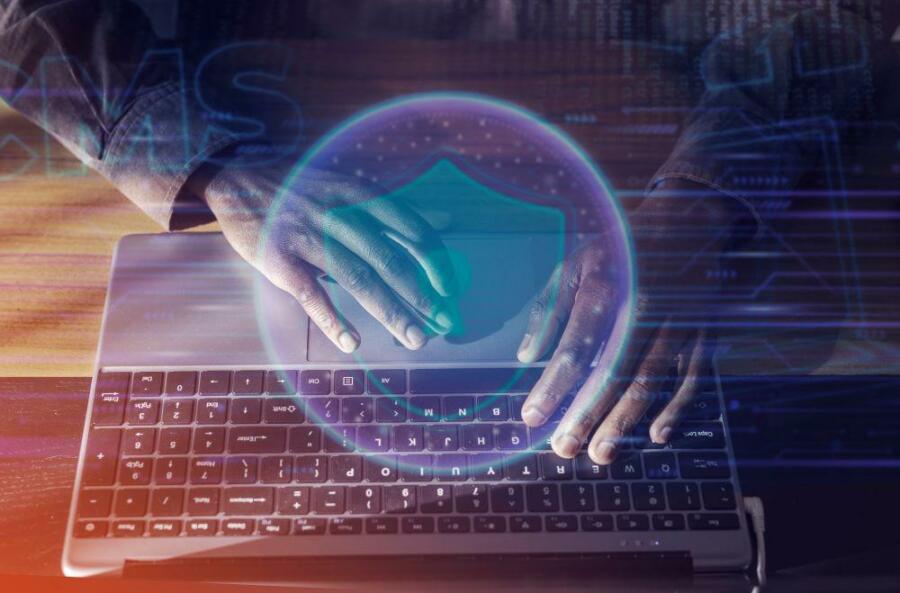
Machine Learning Techniques for An Efficient Fraud Management System
One key aspect of machine learning in fraud management system detection is its ability to employ supervised and unsupervised learning techniques. Supervised learning involves training algorithms on labeled data where fraudulent and legitimate transactions are identified. Through this process, algorithms learn to distinguish between the two categories, enabling them to detect future instances of fraud.
On the other hand, unsupervised learning operates without predefined labels, allowing algorithms to uncover hidden patterns and anomalies within the data. This capability is particularly valuable in identifying new and emerging fraud patterns that have not been previously encountered.
Features and Patterns That Machine Learning Algorithms Use for Frauds
Machine learning algorithms excel at identifying intricate features and patterns in transaction data that might indicate fraudulent behavior. By analyzing historical data, they learn to identify markers of suspicious activity, such as unusual purchasing patterns, geolocation anomalies, or atypical spending amounts.
These algorithms can detect real-time abnormalities, flagging potentially fraudulent transactions for further investigation. Machine learning algorithms remain effective even as fraud techniques evolve by continuously learning and adapting to new patterns. The application of machine learning in fraud prevention extends beyond anomaly detection. Behavioral analytics and user profiling are crucial in identifying fraudulent behavior based on deviations from normal user patterns.
By building user profiles and analyzing behavior, machine learning algorithms can detect anomalies that may indicate fraudulent activity. For example, suppose a user suddenly exhibits behavior that deviates significantly from their usual patterns, such as making large transactions outside their normal spending habits. In that case, the algorithm can flag it as potentially fraudulent.
Advanced Techniques in Fraud Prevention
In the ever-evolving landscape of payment fraud, advanced techniques powered by machine learning offer an enhanced arsenal for fraud prevention. These techniques go beyond traditional approaches, leveraging the capabilities of machine learning algorithms to fortify defenses and stay one step ahead of fraudsters. Let’s explore some of these advanced techniques:
1. Application of Anomaly Detection
Machine learning algorithms excel at anomaly detection by identifying unusual patterns and deviations from normal transaction behavior. By establishing baseline patterns and learning from historical data, algorithms can quickly flag transactions that exhibit suspicious characteristics. This enables businesses to investigate potential fraud cases promptly and take preventive action, safeguarding themselves and their customers.
2. Behavioral Analytics and User Profiling
By harnessing behavioral analytics, machine learning algorithms can analyze user behavior, build comprehensive profiles, and identify deviations from normal patterns. These profiles capture various attributes, such as transaction frequency, spending habits, and interaction preferences. Any anomalies detected in user behavior, such as sudden changes in transaction volume or unfamiliar purchase categories, can serve as red flags for potential fraud, prompting further scrutiny.
3. Real-time Transaction Monitoring and Alerts
Real-time transaction monitoring, powered by machine learning algorithms, ensures immediate response to potential fraud and a good fraud management system. Algorithms analyze real-time transaction data, assessing risk factors and comparing them against historical patterns. If a transaction triggers predefined risk thresholds or exhibits suspicious characteristics, alerts are generated, allowing organizations to take swift action to prevent fraudulent activities.
4. Fraud Risk Models and Scoring Systems
Machine learning algorithms are pivotal in constructing robust fraud risk models and scoring systems. By training on historical data, algorithms identify key fraud-related variables and patterns. These models and scoring systems assign risk scores to transactions based on transaction type, user behavior, and historical fraud patterns.
Organizations can then prioritize their fraud prevention efforts by focusing on transactions with higher risk scores and optimizing resource allocation for maximum effectiveness.
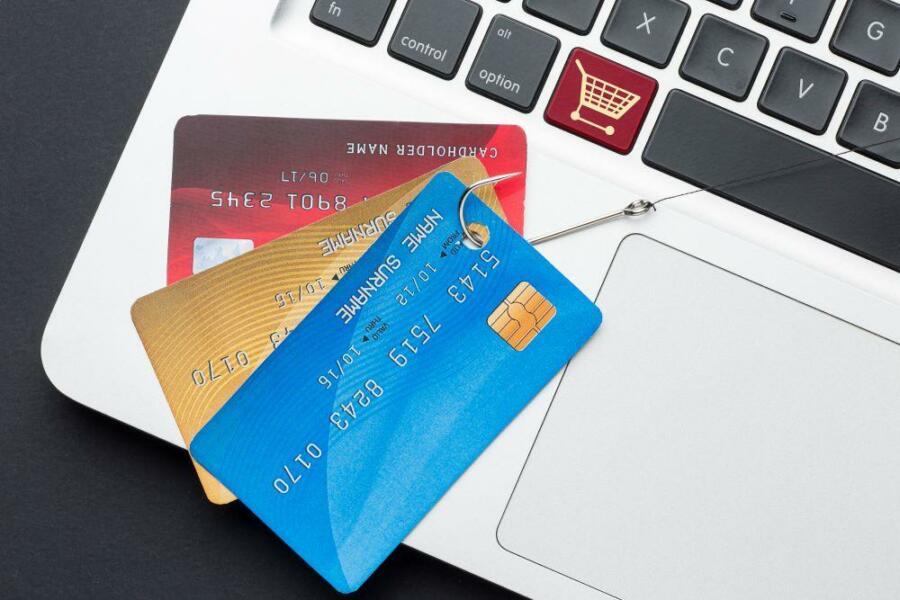
Challenges and Limitations
Machine learning algorithms heavily rely on high-quality data for accurate analysis and decision-making. Incomplete or inaccurate data can hinder the performance of these algorithms, leading to suboptimal credit card fraud detection outcomes. Organizations need to ensure data integrity, conduct regular data quality assessments, and implement robust data collection and cleaning processes to enhance the reliability of machine learning models.
Also read: 7 Ways To Leverage Machine Learning For Automated Health Insights